Interaction and Speaker Role Detection
Context
Work on features extraction and segmentation carried out in our team provides sets of low-level features or segments that can be considered as basic events. Their exploitation and their combination carried out in different ways can lead to the detection of new features or events of a higher or more semantic level. In previous work, we have studied temporal relationships between basic audio and video segments (see TRM page). On the basis of this work and in order to address the issue of conversational speech extraction and processing, which was the core of the EPAC Project, we have considered that conversational speech could be found into sequences where interaction between speakers may occur.
Overview
Using atomatic speaker diarization results as input (see Speaker Diarization page), a temporal analysis based on speaker alternation detection is carried out and produces a set of macro segments called interaction zones as shown in Figure 1.

Figure 1: Macro segmentation into interaction zones, speakers involved (LOC#) and interactivity level.
Our work hypothesis is that the presence of conversational speech may depend on the type of interaction (chronicle, debate, interview…) that can have taken place between speakers, in content like broadcast news or magazines, as well as the role played by each speaker (anchor, journalist, guest/other). To better characterize these interaction zones and enrich them with complementary information, local and global features are extracted: the former to characterize each interaction zone (interactivity level, speakers involved, …), the latter mainly to characterize each speaker’s “behavior”. In this case, three types of features are directly extracted from each speaker diarization results, to form a 36-parameter vector based on temporal, acoustic and prosodic features, representing respectively the speaker activity, the acoustic environment and the way he/she ispeaking.
.png)
Figure 2: Example of temporal information (speech segment repartition) depending on the speaker role (anchor vs guest)
Qualitative observations (see the example in Figure 2) have tended to show that speakers’ behavior may depend on their respective role. This led us to develop a system for speaker role recognition, each speaker being represented by a parameter vector. Figure 3 shows a general description of this system. Our best system, intended for five role classes, including the three main roles used in state-of the art systems (anchor, journalist, other) but making a distinction between punctual and non-punctual speakers (for journalist or other only), is based on a hierarchical approach using SVM classification methods. It has been evaluated on the 13 hours corpus of audio content from the ESTER2 evaluation campaign, reaching about 82% of well recognized role, which represents 89% of the test corpus correctly labelled in role (see the main publications for details). This evaluation shows that anchor (92%) and punctual speakers ( journalist 86% ; other 89%) are particularly well recognized.

Figure 3: General overview of our Speaker Role Detection system
Applications
These good performances allowed us to use speaker role recognition results in content structuring application. The first structuring level consists in proposing a first macro-segmentation results based on anchor coverage to make a distiction between presented units (macro segments with at least one anchor) and breaking units (macro segments without anchor). The second structuring level consists in characterizing more precisely interaction zones, by considering the roles of each speaker involved and defining rules to make a distinction between different types of interaction (interview, debate, chronicle or handing over sequences).
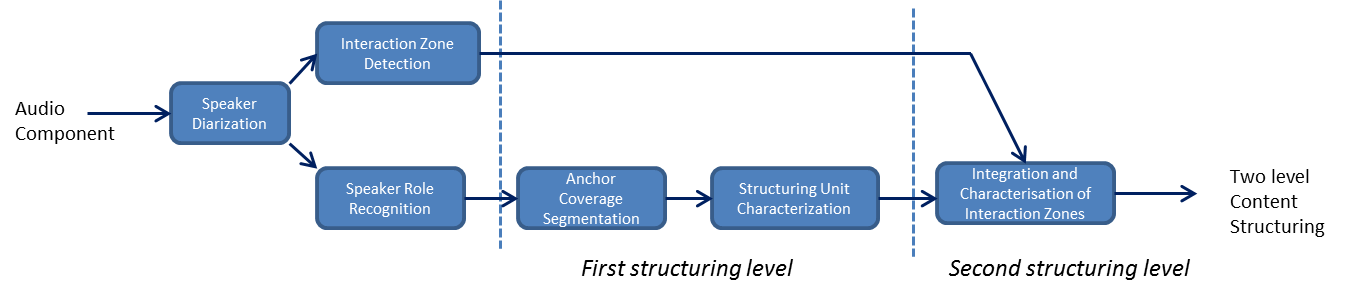
Figure 4 : Two level structuring process
This process described in Figure 4 produces a two level structuring result based on the audio component only, as illustrated in Fugure 5.
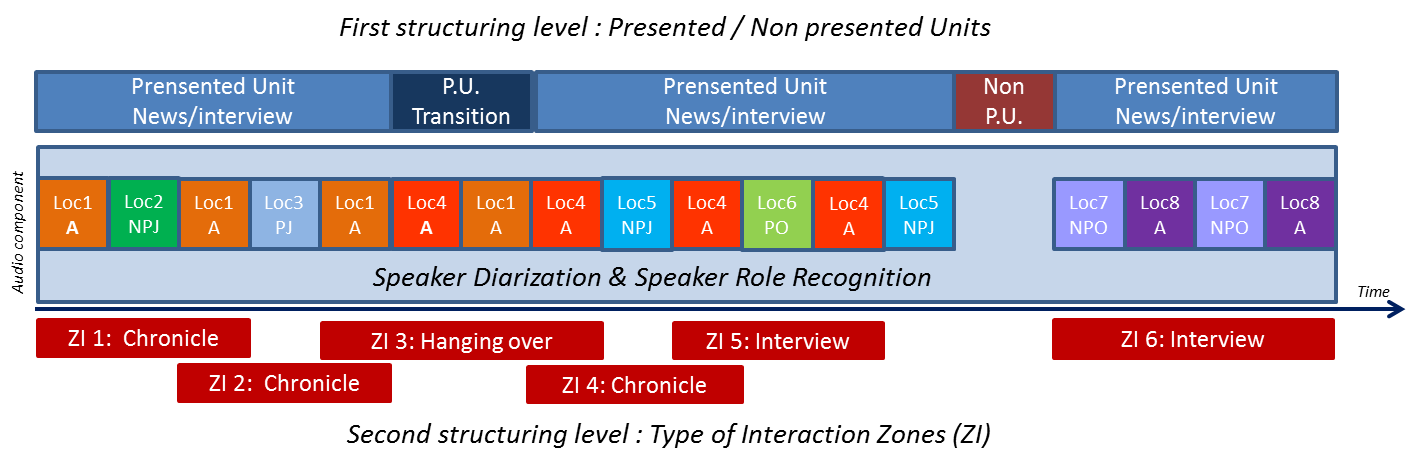
Figure 5: Using Speaker (Loc#) Role Recognition results (A for Anchor, J for Journalist, O for Other, NP for Non Punctuel, P for Punctual)
in a two-level structuring approach. Example of results.
This work has been the core of Benjamin Bigot’s. PhD entitled “Searching for stakeholder roles and their interactions for structuring audiovisual documents” (available in French only).
Projects
- This work was conducted within the EPAC Project (2007-2010)
Contributors
- Benjamin Bigot
- Isabelle Ferrané (contact)
- Julien Pinquier
- Régine André-Obrecht
Main publications
Benjamin Bigot, Isabelle Ferrané. From Audio Content Analysis to Conversational Speech Detection and Characterization. Dans : ACM SIGIR Workshop: Searching Spontaneous Conversational Speech (SSCS 2008), Singapore, 24/07/2008, ILPS (Information and Language Processing Systems), p. 62-65, 2008.
Access : http://ilps.science.uva.nl/SSCS2008/Proceedings/sscs08_proceedings.pdf
Benjamin Bigot, Julien Pinquier, Isabelle Ferrané, Régine André-Obrecht. Detecting individual role using features extracted from speaker diarization results. Dans : Multimedia Tools and Applications, Springer-Verlag, Vol. 60 N. 2, p. 347-369, septembre 2012.
Summary Access : http://dx.doi.org/10.1007/s11042-010-0609-9
Benjamin Bigot. Recherche du rôle des intervenants et de leurs interactions pour la structuration de documents audiovisuels. Thèse de doctorat, Université Paul Sabatier, juillet /july 2011 (French)
Abstract URL : http://tel.archives-ouvertes.fr/tel-00632119/fr/ ftp://ftp.irit.fr/IRIT/SAMOVA/TH/manuscritTheseBigot2011.pdf