Spectral Cover
Context
The analysis of instrumental activities of daily life is an important tool in the early diagnosis of dementia such as Alzheimer. The IMMED project investigates tele-monitoring technologies to support doctors in the diagnostic and follow-up of the illnesses. The project aims to automatically produce indexes to facilitate the doctor’s navigation throughout the individual video recordings. Water sound recognition is very useful to identify everyday activities.
Indeed, the automatized recognition of water flow events may enable the indexing of several dailylife human activities, such as: activities of hygiene (e.g., hand-washing, teeth brushing), diet activities (e.g., cooking, making coffee), housework (e.g., doing the dishes, moping, cleaning), and leisure (e.g., gardening). Consequently, different studies dealing with water flow recognition have been presented in the last years. Some of them support aging in place by monitoring an elder’s activities of daily living. Other studies aim at specifically identifying water sounds in bathrooms to obtain behavioral information on activities of personal hygiene with respect to privacy.
In this domain, the major part of the studies follow a typical recognition scheme that computes acoustics descriptors on short frames of the signal, thus composing patterns from the different events to recognize. Descriptors are usually extracted from the temporal domain (energy, zero-crossing rate, etc.) or from the spectral domain (spectral centroïd, spectral flux, spectral roll-off, etc.), among them, the Mel Frequency Cepstral Coefficients (MFCC), which come from speech recognition systems.
These approaches has been tested on the IMMED corpus, without achieving satisfactory results. Indeed, the main difficulty of our corpus is that water noises are overlapped with other noises and with speech. Another difficulty lies in the heterogeneity of data collected from different places.
We can see on the following figure, an extract of 5 minutes composed by a long water flow event: a speech part overlaps the end of the water flow event and its amplitude is very high in comparison to the other parts. Lot of noises are present during all of the excerpt, mainly noises from the kitchen activities. We tested different low level descriptors on this excerpt: temporal features (energy, energy per band and ZCR) and spectral features (centroïd, spread, skewness, kurtosis, roll-off, flux, variation coefficient, and flatness). The figure shows the most representative features: the ZCR, and the spectral the spectral flatness. This two curves are impacted by the presence of the low frequencies of speech.
.jpg)
Overview
We introduced a new feature that we call spectral cover because it reacts to large spectral band sounds like high colored noises, even in presence of speech. The spectral cover is given by:
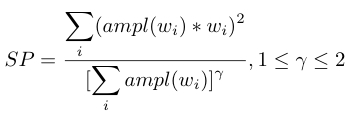
where wi represent the frequencies and ampl(wi) their amplitudes, from the Fourrier transform. If we compare the spectral cover with the well-known spectral centroïd given by:
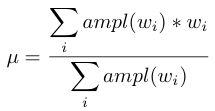
An important difference is the introduction of a power in the numerator: it may boost the high frequencies, in comparison of the spectral centroïd or the spectral spread. An other difference, the power γ, makes our feature sensitive to the absolute signal level, unlike spectral centroïd. The γ parameter allow to tune the sensibility of the feature to the signal level.
Application
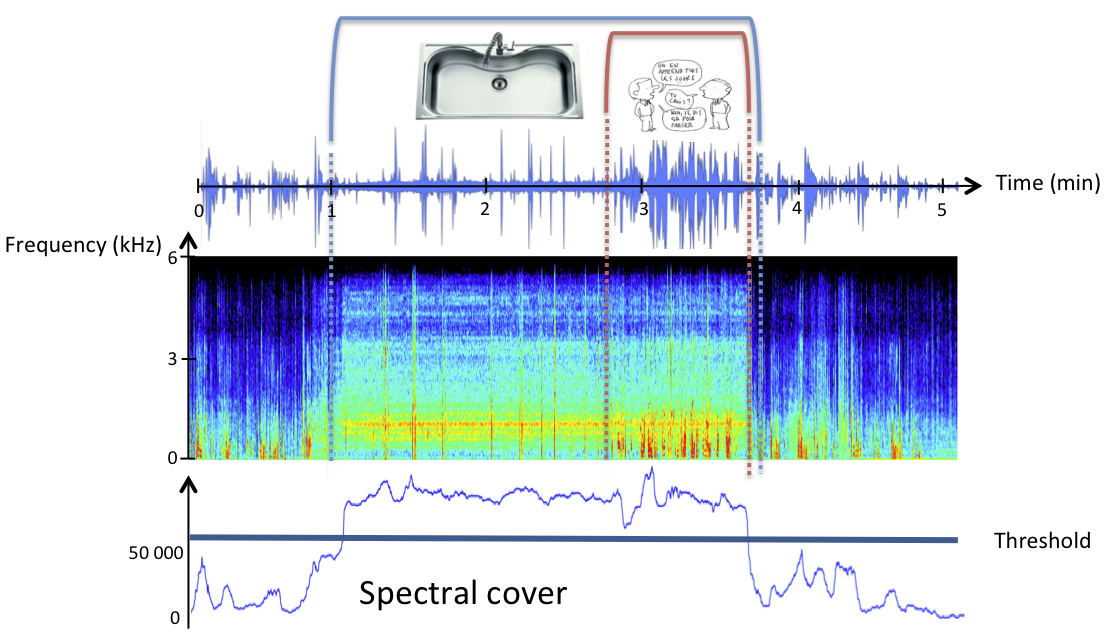
We can see on the following figure that the spectral cover stays almost constant during the water flow event even in the presence of voice. It becomes lower outside the water flow sound event, that’s why on this extract we can separate easily the water flow event with a simple thresholdA system based on robust thresholds was build to detect these water flow events. This system was assessed on IMMED corpus: more than 7 hours of real life records. The results are very satisfying regards to task difficulty. Moreover, this system outperformed the state of the art approaches. Finaly, the spectral cover is a very good feature to detect water flows event. Furthermore, it has been designed to detect noisy sounds with high frequencies. Thus, it could be easily used in another system to detect other sounds, for instance vocal fricatives.
Projects
- IMMED Indexing Multimedia Data from Wearable Sensors for diagnostics and treatment of Dementia.
Main publications
Patrice Guyot. PhD Thesis. Caractérisation et reconnaissance de sons d’eau pour le suivi des activités de la vie quotidienne. Une approche fondée sur le signal, l’acoustique et la perception.
[Guyot2013] Patrice Guyot, Xavier Valero Gonzalez, Julien Pinquier, Francesc Alias. Two-step detection of water sound events for the diagnostic and monitoring of dementia (regular paper). Proceedings of the IEEE International Conference on Multimedia & Expo (ICME 2013), San Jose, USA, july 2013.
[Guyot2012] Patrice Guyot, Julien Pinquier, Régine André-Obrecht. Water flow detection from a wearable device with a new feature, the spectral cover. Proceedings of the IEEE International Workshop on Content-Based Multimedia Indexing (CBMI 2012), Annecy, France, june 2012.